Amsterdam
Team Members
Marie Blønd (presenter) - Tine Schjaerff Soerensen (presenter) - Vimviriya Limkangvanmongkol (presenter) - Juan-Carlos Goilo - Min Sheng Qian - Natalia Ishchenko
1. Introduction
Using the DMI-TCAT toolkit (Borra & Rieder, 2014) for topic-based sampling (#Amsterdam) we are provided with a dataset for social analysis of the Amsterdam tweets or conversation topics enabling a geo-location demarcation within the periphery of Amsterdam. Suggestions of using the 1% of Twitter data for network analysis has shown that the results of random sampling and geo-tags may infer population representation and that use practices of hashtags has increased (Gerlitz & Rieder, 2013). Taking this further than handling big data using geotags, our ambition is to reflect upon the topical use of #(city) twitter samples and argue for the possibility of appropriate valuable social data and narratives of on-location events and concepts to be mined, analyzed and presented in intriguing ways (Crampton et al., 2013). Conducting this case-study in June 2014, it became obvious that Twitter was hi-jacked by the World Cup 2014 requiring an explorative filtering process to find other significant issues. Applying a bottom-up data mining process in order to find the appropriate data underpinning this through qualitative text and image data available in (re)tweets, we aim to situate on-ground events and local concepts. In other wods, what is happening on-location according to Twitter for #Amsterdam?
1.1. Research Question(s)
How can a remote analysis be conducted with the aim of finding on-location appropriate data using (#cityname) topic-sampled data and how are these locally situated and translated globally?
- Who is speaking about (remote) and from the city (geo-on-location)?
- What is the city speaking about in terms of emerging events and locally translated concepts?
- What are the on-location local issues and how do they tie back to global movements/trends?
2. Method
With an offset in the DMI-TCAT database with the topic-based hashtag #Amsterdam we were faced with a dataset of over 2 million tweets over a period of approximately a month. Handling this pre-existing data for a remote analysis of events and emerging concepts, this case-study evolved into an
exploratory data analysis approach (Tukey, 1977) reflecting on what we methodologically have termed a bottom-up approcach. This appoach focuses on qualitative rather than quantitative data by reverse (up/down) scaling within the dataset, filtering hijacking themes and demarcating queries according to evolving topics (#) and geotags. Limited by the small amount of geotagged tweets we have also included other indicators of location such as user-language settings and user-defined location, although this geo-accuracy may also be questioned.
Step 1: The query dataset was demarcated with the hashtag #Amsterdam (amstardam, amsterdam, amsterdama, amsterdamas, amsterdão, amszterdam, Άμστερνταμ, Амстердам) summarized to more than 2 million tweets collected during 21st of May to the first week of July 2014. To overcome the vast dataset with a qualitative assesment, it was decided to scale-down (take a random sample) to look at life in #Amsterdam in one day. Findings included that during June 2014, tweets with #Amsterdam were hijacked by major global events, especially the World Cup 2014. Furthermore it was dominated by branding and marketing campaigns mostly wthin the sex and beer industry.
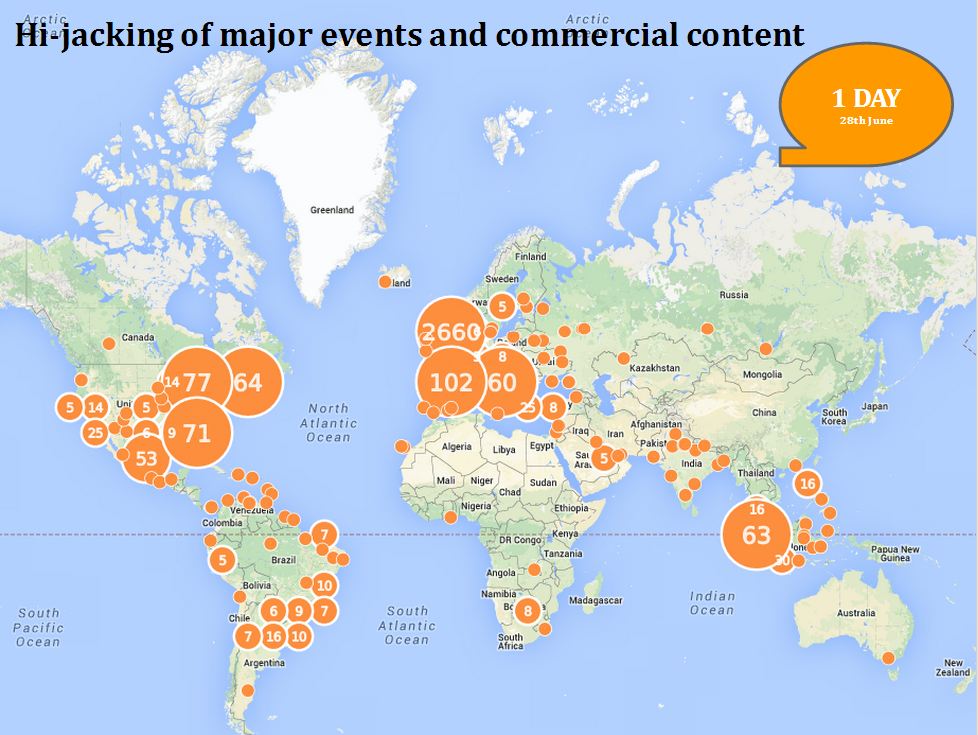
Step 2: Scaling up to focus on most frequent hashtags(#) in the sample dataset for one week (during 23rd to 29th of June 2014) with geolocation we qualitatively came across top 50 tweetsthat again was hijacked by the bots and brands in the aspects of tourism, sexcam, transportation and the World Cup 2014-related.
Step 3: We attempted to exclude the hi-jacking themes related to the World Cup 2014 or commercial campaigns related to sex, jobs and beer. This list was thus filtered reveiling a # list for further study, where we approached the list qualitatively and found interesting hashtags closer to the bottom of the list. These were among other hemes limited to (1) #participatie and (2) #zwerfie for further study.
Step 4: Scaling up within the dataset (for the month of June 2014), these topics according to geo-location we made a search query for these 2 themes and their co-# to situate the tweets/conversations. The full map can be viewed here
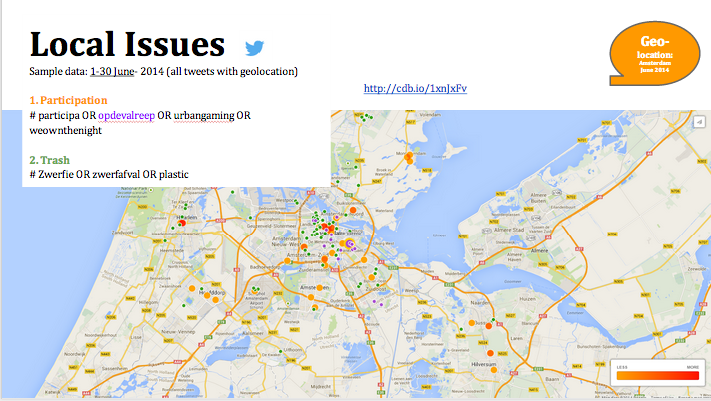
2.1 Methodology: Participation (#participa )
Further queries into the #participa revealed a significant mention of #opdevalreep or #valreep which was further explored with content-analysis on Twitter and thick data (Wang). Futher investigation revealed that this topic was a reflection of a (spontaneous) on-location event in Amsterdam in a place called Valreep (plotted in on the previous map) or rather a 'clash' between the squatting community and the authorities (police) concentrated within the timeframe of 15th-20th June. Scaling in on this period it enabled us to go through the tweets and extracting the conversations into a narrative of the authorities evicting the building and the squatters resistance.
Scaling in on this period it enabled us to go through the tweets and extracting the conversations into a narrative of the authorities evicting the building and the squatters resistance by using wordfrequency query and presenting these visually on a timeline, density of the bars reflecting frequency of mention. Elaborating this timeline with images and tweets a narrative appears of the temporality of the event.
2.2 Methodology: Trash (#zwerfie)
Step 1: We started from the #Amsterdam dataset tweets with #Zwerfie (a combination of 'selfie' and 'zwerfafval', which is the Dutch word for litter). The Dutch national campaign ask people to pick up one piece of litter a day, make a 'zwerfie' and post the photo on Twitter with #zwerfie added. As the campaign mainly encourages non-plastic use and clean up public space, we used
zwerfie OR swarfafval OR plastic as major keywords for searching queries on TCAT. We selected to only look at all tweets within one-month period (from 1st 30th June 2014) with geolocation. We finally collected 145 tweets.
Step 2: With the limitation of small numbers of tweets, we decided to focus on the quality of data. Therefore, we have qualitatively explored the data set by reading all of the collected tweets. Within the data set of one-month period, besides zwerfie movement, we found another two salient movements that people most tweeted about by using #zwerfie.
Zeroplastic Week (9th - 15th) and
Clean Up were very relevant for the trash theme in terms of local events which are tied to the global parts.
Step 3: We created our coding scheme from three movements:
1. Zwerfie; 2. Zeroplastic Week; and 3.Clean Up. We deleted spammed and irrelevant tweets. Therefore, seventy tweets were the final unit of analysis which we coded manually whether their content was related to one of the three categories as follows:
1. Making picking up the trash fun - Tweets were mainly relating to the Dutch national zwerfie campaign that Dutch residents and tourists were asked to pick up one piece of litter a day, make a '
zwerfie' and post the photo on Twitter with #
zwerfie added. The campaign mainly aims to create the awareness to make the environment clean and green.
2. Zeroplastic Week - Tweets were mainly related to a week (9th - 15th of June) during which people were asked to stop using new plastic objects. The international campaign aimed to initiate the awareness to use more of green objects for people's needs and respect the environment more. The campaign was participated by people among 20 countries around the world, including the Netherlands.
3. Keep the canal clean - Tweets were mainly related to cleaning up the canal in Amsterdam. The campaign is a part of worldwide Clean Up campaign aiming to make the communities clean and conserve the environment.
3. Findings
3.1 Participation /Valreep
3.2 Zwerfie/Trash
- As for the trash issue, #zwerfie is a hashtag that connects subtopics and related topics concerning sustainability together. The visualization shows that not only on-location campaigns, such as zwerfie but also global ones, such as Zeroplastic Week and Clean Up are intervowen in the online conversations under the major theme related to trash. As such, #zwerfie is one of the significant hashtags that allows Twitter users to initiate conversations from #Amsterdam about the city. Interestingly, such conversations are shared by many users who synchronously perceive Amsterdam in a green way and connect Amsterdam with the global sustainability movements
4. Conclusion & further study
Our findings from an experimental approach to mining appropriate data in a topic-based sampling emphasizes that the filtering process may improve from use practices such as up and down scaling in data, excluding top hijacking words and hashtags as well as co-word analysis. Another level of importance in the methodology is the assessment of local issues to ensure that the topics are tweeted on-location. Using these practices simultaneously as a 'botom up apprach' in an inductive manner, identifying local keywords and hashtags and applying it to a larger dataset can present intriguing insights into how events and movements take place and develop on-location. Local issues could in the case of participation be built into a temporal narrative based on semantic analysis, highlighting tensions and contradictions in handling grass-root community spaces versus official city branding. This is for example shown with The Valreep receiving award for the most creative social project and conferences on Amsterdam mentoring Athens on making participation effective in diverse neighbourhoods while the authorities (police) evicts the resisting squatting community from the building with the purpose of tearing it down.
The topic of Zwerfie (trash and selfie) has with this approach suggested that coding of the data may be used for insightful findings, as in this case it shows that a local translation of a global movement takes place on-location in Amsterdam. This movement is locally situated in one of the greater parks in Amsterdam and has sub-movements involving the notorious canals of the city. The topology of adding #Amsterdam to the tweet becomes enacted by the citizens in a form of gamification and ties into global movements. Global digital phenoma such as selfie and global digital initiatives as Zeroplasticweek are being reshaped locally by Amsterdam citizens and translated into the Dutch concept Zwerfie.
Proposing with this case-study that hashtags can become an empowering Twitter tool for local communities and movements within cities, driven by the citizens and grass-roots, to enlighten the public could be suggested for further studies. Furthermore the motivation driver for citizens through concepts of gamification or personification could also be explored further as a motivator for a participatory public on Twitter.
Bibliography
Borra, E. & Rieder, B. (2014) Programmed Method. Developing a Toolset for Capturing and Analyzing Tweets, Aslib Journal of Information Management , 66(3), 2014, 262 - 278.
Crampton, J.W. & Graham, M. et al. (2013) Beyond the geotag: situating big data and leveraging the potential of the geoweb, Cartography and Geographic Information Science , 40(2), 2013, 130-139.
Gerlitz, C. & Rieder, B. (2013) Mining One Percent of Twitter: Collections, Baselines, Sampling, M/C Journal , Vol. 16(2), May 2013. <http://journal.media-culture.org.au/index.php/mcjournal/article/view/620>
Tukey, J.W. (1977) Exploratory Data Analysis#. New York: AddisonWesley.