Detecting the socials
Team members
Team Users & Interactivity: Rasha Abdulla, Davide Beraldo, Augusto Valeriani, Navid Hassan, Tao Hong, Johanne Kuebler, Carolin Gerlitz.
Team Bots: Evelien D'heer, Bev Skeggs, Wifak Gueddana, Esther Weltevrede.
Team Hashtags: Diego Ceccobelli, Catharina Koerts, Francesco Ragazzi, Andreas Birkbak, Simeona Petkova, Noortje Marres.
Design Team: Gabriele Colombo, Stefania Guerra, Carlo de Gaetano.
Tech Team: Erik Borra & Bernhard Rieder.
Introduction
New media research has paid attention to empirically inquire into the medium-specificity and the happening of issues in the context of social media. The affordances of medium-specific features, both as research objects but also as methodological devices have been subject to various research projects in software studies, digital method and issue mapping. Whilst there are numerous attempts that focus on or deploy media or issue dynamics empirically, the notion of the social in platforms has not been subject to such empirical scrutiny. In the context of social media, we suggest, the social is more often being proclaimed, being taken for granted and thus increasingly rendered opaque. This a priori social can be situated in features (possibilities to interact and to connect), content (which invites for spreading), context (off and online distances and location) or social meta data (such as friend and follower connections and countable expressions of appreciations though Likes or Favourites). But instead of taking the social for granted, we rather follow Emma Uprichard who contest: Just because it is called social, doesnt make it social!
Overall Research Question:
How to empirically detect and describe the social in social media (twitter) data?
The aim of this research project is thus to abstain from an a priori or taken-for-granted social and empirically approach whether we can detect the social in social media and whether it is accomplished in the first place at all. The social, we suggest, cannot be merely proclaimed or situated in the material-technical infrastructure of platforms, but can come in various forms, and can also fail. The central research question emerging from this approach is: How can we empirically detect and describe social and non-social dynamics? As we still seek to tie this question to a focus on the medium, we also ask: How is the social accomplished within social media? What are possible descriptors to empirically account for the social? And what forms of social(s) do emerge in this empirical approach?
Trying to empirically detect the social, one might argue, requires an a priori account of what kind of social we are actually looking for. Is it a Durkhaimian, a Weberian or a more Deleuzian account of the social? In the context of this project we decided to abstain from a predefined definition and instead draw on an emergent account of the social - taking departure from the various perspectives we developed on the social, the practices we encountered and the debates we had about what we consider as social and what not? As a consequence, rather than fining ways to detect the social, what we found was various socials.
Data set
To operationalise this empirical investigation into where, how and in what form social dynamics are being enabled and take place in social media, we decided to focus on the platform Twitter and a dataset on the topic of privacy, captured with the Digital Methods Twitter Analysis and Capture Tool (TCAT):
Dataset on privacy (keyword search)
Interval: 23 May 2013 - 15 June 2012
Number of tweets: 919.234
Users: 482.195
Twitter is of interest to us in this pilot investigation as it allows for a limited number of medium-specific activities and thus comes with a various yet not overarching amount of possibles measures. The topic based sample on privacy allows to address a topically varied sample informed by both political and news events, including prism and the chase for Edward Snowden, but also contain bot activities, everyday chats and the inevitable resonance of celebrities. Although the original privacy dataset contain a much longer time interval ranging from November 2012 until end of June 2013, we had to limit the interval to keep the work doable in terms of technical support and allocated time in the framework of the Digital Methods Summer School.
Approach:
Within the pilot project we followed three entry points to the data deploying and thus testing a variety of measures, ranging from an actor, medium and content perspective.
1. Users: Detection of user based activity and interactivity patterns.
2. Bots: How can non-human actors be identified in Twitter data, how present are they and how do they inform or co-produce sociality?
3. Hashtag Profiling: How can we detect the sociality of content dynamics and what are social hashtags?
A further aspect of our research objective was to explore how different metric, measures and descriptors allow to approach and translate sociality differently. The majority of measures used across the subgroups draws on medium-specific features of the platform, such as retweets, @reply chains, metadata or hashtags and contained a further interest in the variation and dynamism of these possible descriptors of the social over time.
I. Users & Interactivity: Activity Patterns
Introduction
One pathway to identify ways to empirically study the social are users and their activities. In our attempt to focus on emergent notions of sociality (in its plural form), as well as possible empirical descriptors, we traced the actions and interactions of users involved in the Twitter set to map out and account for forms of social life. Due to the large amount of users active in the dataset, we soon encountered problems of scale as focusing on the most active, most talked to or most retweeted users presented us with rather specific results - which are difficult to consider very social at all.
Research Questions:
1. What patterns of activity & interactivity can be detected?
2. How to study social life by starting from users & activities?
3. What forms sociality did we detect?
Method
The methodological approach of studying user activities focused on a variety of measures.
In a first step, a general overview of the dataset including the use of URLs, Hashtags, Mentions and RTs was conducted. Zooming in on the most active and most mentioned users, the top 20 list of each categories are juxtaposed and the users categorised. Focusing further on the engagement of users with specific hashtags, a bipartite hashtag-user graph has been prepared using TCAT analytics and Gephi. Here, the hashtag privacy is deleted as the term is the search term for the overall sample. Again, the focus was placed on the most frequently used hashtags among users.
In a next series of steps, the project moved away from the top frequencies to investigate the middle grounds. Doing so, we identified a series of users which are at the same time active but not most active (100-300 tweets in the dataset), but also receive at least 20 @mentions. We visualised the distribution of tweets and mentions of these 120 users to identify (inter)activity patterns. Further we compiled an mention graph for the selected users with Gephi to visualise the interconnectedness of their interactions and a bi-partite hashtag-user graph to compare to the most frequently used hashtags above.
In a further series of steps, the focus was placed on conversations approached through @reply chains. Here, the focus was places to identify reply and conversation patterns and bring them together with user activity and user categories. A new feature of the TCAT tool allowed to trace reply chains, unfortunately it was difficult to detect the root tweet from which conversations originated. A series of conversational categories as well as there frequencies were identified emerging and user categories were added to this.
Another section of the sub-group gave attention to retweets. The temporal distribution of retweets and their frequency were plotted on a timeline, bringing to attention the distraction of top frequency retweets. Retweets were identified here by using the identical tweet analytics of the TCAT tool. However, one has to outline the limitations of this metric, as it does not take the variety of possibilities to create retweets, such as modified tweets, into account. To identify which content resonates within the sample, the top 10 retweets per day were categoriesd and the frequency of retweet categories over time was visualised. Again, the focus on top frequencies posed a series of problems as discussed in the findings section below.
Findings
Exploring the dataset and general measures of user activity, we find the typical long tail pattern of participation. 75% of all users in the dataset participate with one tweet and less than 1% participate more than 100 times. Compared to a pilot study on random Tweets samples, the privacy sample comes with a relatively high proportion of tweets with hashtags (almost 50%), whilst only a fourth of all tweets contain hashtags. More than half of all tweets, 61.5% contain mentions and thus address other users and 41.95% contain identical tweets, a specific way to create a retweet.
In a first step we zoomed in on the 20 most actively tweeting and 20 the most mentioned users, which we categorised based on their profession, activities or topical focus. Among the most interesting findings is the high presence of bots in the active user group as well as the high discrepancy between active and mentioned users - users either produce many tweets or are mentioned a lot, but a balanced distribution cannot be found in the top ranges. Whilst bots are most prominent in the active user group, both in number and in the amounts of tweets they generate, we can also find few technology sites, general news outlets, bloggers and news outlets focused on privacy concerns. In the most mentioned group, individual journalists, news outlets, and other public figures from music, entertainment, politics and NGOs can be found, some of which have many followers too.
Exploring the top frequency levels further, the bi-partite hashtag-user graph shows a very interesting finding, as we see too almost disconnected clusters of hashtags (green nodes), connected to by users (grey nodes) deploying the hashtag. The left cluster contains a topically diverse set of hashtags. From the most frequently used event based hashtags such as #nsa, #surveillance and #prism in the centre, to specific IT companies, social media platforms, to general connections in US politics (#tcot, #p2), the left cluster also contains various astrology signs at its fringes. The right cluster however, stands out through its homogenous density focused on rather generic hashtags such as #photos, #websites, #mp3, #blog, #free or #videochat. The even connections between these hashtags and the users suggests that we have encountered a retweet peak, which later proved to be the case: The cluster was caused by this tweet: Spam RT @mysafemedia: [#FREE #WEBSITES+#BLOG
http://t.co/vZdqEruI89] Upload #Photos/#Videos/#MP3/CustomForms/Live #VIDEOChat/#Privacy/#EmailBlasâ¦
http://www.mysafemedia.com which gained more than 10.000 - and also poses the most frequently retweeted tweet of the entire set.
The main finding from these initial explorations was that activity and interactivity are not balanced when focusing on the top frequency tier. Instead, what we find are dynamics specific to the medium, such as retweet peaks and a strong presence of bots. Hence, we developed an interest to shift attention towards the middle frequency levels, to explore the activities of users who are active and interactive at the same time. After identifying a set of 120 both active and interactive users, we detected the following: the medium range has a much more balanced distribution of tweets and mentions, with major overlaps between both. Among these users are barely any bots and news outlets but mostly activists of all sorts. However, when bots were part of this group, they posted on-topic content actually related to privacy and security issues and thus received mentions as well.
Looking further into the user-mention graph, a central, rather dense cluster of mutually mentioning users emerge, accompanied by rather few outliers that are not mentions a lot by others and vice versa. We further explored the engagement with hashtags in a bi-partite hashtag-user graph and found that compared to the graph focusing on the most frequently used hashtags, the focus is more on politics and eventive concerns here, ranging from the centrality of #nsa, #prism, #surveillance, to general US politics such as #tcot, to #infosec, #occurpy and a variety of IT companies. The particular pathway from top tier to medium frequency suggests to us that different entry points allows to explore different socials. Whilst the top tier is very much driven by automated activity (bots) and the driving force of offline-authority of celebrities and news outlets, the middles ground allows a glance on different forms of interaction and topic attachment.
In a next step, we focused on emerging conversations within the dataset which were approached by looking at @reply chains. The visualisation below shows an overview of the reply chains found in the dataset. The bigger a node is, the more followers the user writing the corresponding tweet got. Most interestingly, the size of conversations seems rather evenly, without extreme ends. As documented in the next visualisation, three conversational formations emerge. First, we encountered the chain, a mutual reply network of successive replies. It makes up the least proportion of all conversations. The most prominent conversational type is the star, where various users respond to a root tweet without receiving further response. A mix of both and other types makes up the third and second largest groups in which a root tweet generated responses of which some are further replied to and others not.
When looking at the length of the reply chain, we see that the vast majority only comes with 2 tweets, that is one reply. Only a fraction of reply chains exceed the length of 10 tweets, suggesting that we are dealing with a large amount of one-off responses and a variation of actual reply and interaction chains involving multiple answers or users.
Exploring the users involved in these reply-chains further, we noted differences in follower counts for the different conversational patterns. Star-like conversations often come with a discrepancy in follower count, as the user of the root tree has many followers, the respondants have much less. In chains and other forms the number of followers is more similar, so that interactions are more likely to occur. From this insight, a we created a tentative categorisation of users. So called attention hubs receive many reply but do not necessarily respond to them whilst having a large amount of followers. Attention seekers, so our assumption, have a high response rate (out degree) but rather little followers. Those who are engaged in actual interactions, generating both content and responses, have a relatively balanced outdegree and follower count. We conclude from the study of reply chains that we need to differentiate between resonance, response and interactivity. Whilst some users, due to their amounts of followers or their offline popularity generate a large amount of responses or resonance for their content, these responses not necessarily lead to further replies or interactions. Interaction, we contrast, can be found among users with similar standing in the community, in which longer reply chains emerge and replies are more likely to be met with further replies.
Our final step was concerned with retweets. Again, we started by looking into the top tier, identifying, categorising and visualising the top retweets. Departing from the difference between resonance and interaction, we were interested what content actually resonates and which aspects of the broad issue of privacy it was concerned. The visualisation below shows the top retweets per day and their frequency over time. The first finding was that the majority of retweeted tweets were retweeted less than 50 times, with few outliers. The most prominent outlier is the aforementioned (spammy) tweet using various media-related hashtags. The content of the top retweets was heavily informed by the time when prism was revealed. Before prism, the top retweets were dominated by highly twitter-specific issues, such as Justin Bieber (who was present in the top retweets every day), as well as other celebrities. After June 07, the topical focus moved to prism related issues and the frequency of the top retweets increased in general, opening up the - yet to be studied - question, whether more general retweets relating to Justin Bieber still prevailed and just moved out of the top 10 daily retweets.
The three subsequent visualisations take the topical category of the retweet into account. The first visualisation shows the above mentioned topical shifts of retweets focused on celebrity and tech news towards anything prism related, involving news, but also analysis, jokes and critique. Interestingly, just as in the bi-partite hashtag-user graph astrology related topics feature strong in the retweets before prism. The second visualisation shows retweet categories organised by the number of retweets within each category. Here, prism jokes and sarcasm feature more prominently than NSA jokes, followed by others (the single off-topic retweet peak), celebrity, prism advocacy and prism jokes.
Conclusion
The focus on user (inter)activity measures has allowed to both approach, but also contest and reflect on what the social in social media is actually constitute of.
1) Methodologically, our findings have resulted in a substantial critique of frequency. Especially in larger datasets with almost half a million users and a million tweets, focusing on the top frequencies seems to be a tempting and even obvious at the start. The sole focus on the top tier, however, only allows to access to very specific practices and what we have found here was not always interactive, but spumy, partially off-topic and dominated by bots. The social we found in the most frequent activities, is a specific one, driven by resonance and automated bot networks of the medium. Yet, it is difficult to disentangle these various socials from the associated media-dynamics, as the top ties is heavily informed by medium-specific retweet and reply peaks and the presence of bots.
2) As a consequence we suggest that research into larger datasets and an interest in detecting the various socials of a debate needs to develop methodological approaches to study medium-levels of activity. Whilst we have found a rather vital middle, with various forms of interaction, the top tier was more oriented towards broadcasting and the low end characterised by one-off participation in the issue. Mutual action and interaction or chain like conversations are more likely to happen among users that do not occupy the top and who have more equal number of followers.
3) Looking into the various descriptors of the social, the question emerged what is the difference between resonance and interaction? Does resonance demarcate a different social than interactive conversations? Do we value one as more social than the other and if - why?
To give consideration to the role of bots and automated communication in the dataset, the second sub-group turn their attention to the detection thereof.
II. Detecting Bots: Coming to Terms with the Botty Social
Introduction
A second pathway to identify ways to empirically study the social is by turning to the non-human: bots. In our focus on detecting emergent forms of sociality, we sought come to terms with the botty social by developing a social method to detect bots. With 'social method' we mean to detect bots by focusing on their habits, patterns of behaviour and their actions and interactions to map out and account for forms of botty social life. We developed four techniques to detect bots
Research Questions:
1. What is the signature of a bot?
(2. How social is the bot?)
Method
The methodological approach is twofold and includes steps for studying 1) the signature of bots and 2) how social they are. The difference between what is a human tweeter and what is a botty tweeter is by no means straightforward as there are for instance human tweeters that behave like bots or users that are bot-assisted. Therefore in a first step we sought to list indicators to identify bot behaviour:
- self-declared bots (search bios)
- analysing the pace, sequence of tweets
- analysing the syntax of a tweet
- presence of one single hashtags
- presence of trending topics
- tracing the source of a tweet (e.g. from web, from mobile, from ITTT, from Tweetadder)
- users with too large following list and/or little followers
From this list we developed four techniques to detect the signatures of bots by analysing the traces of their behaviour: 1) analysing the pace of tweeting, 2) analysting the commitment of a bot to a hashtag, 3) analysing the frequency of tweeting and 4) the source the tweet came from. The first results of the techniques are described in more detail below. In the second step - future research - we seek to qualify the social created by bots in a series of steps. The botty social is qualified by analysing platform specific forms of sociability (e.g. nr of followers, rts, @replies, mentions), analysing the issue dynamics of bots with the asociational profiler, and analysing the shapes of the conversation networks of bots. To get to the social of bots we will compare the results to human users and/or to another data set (climate change, #egypt).
Findings
The first social bot detection technique focuses on the pace of tweets posted by a specific user. The technique uses the Cascade module from the TCAT. In the figure below usernames are listed vertically and dots representing tweets are plotted on the horizontal line. The red dots are the traces of bots which are charactarised by a regular and/or high pace. In addition, we found clusters of bots that automatically retweet tweets, which is visible by the regular blue lines (retweets) in the right bottom corner.
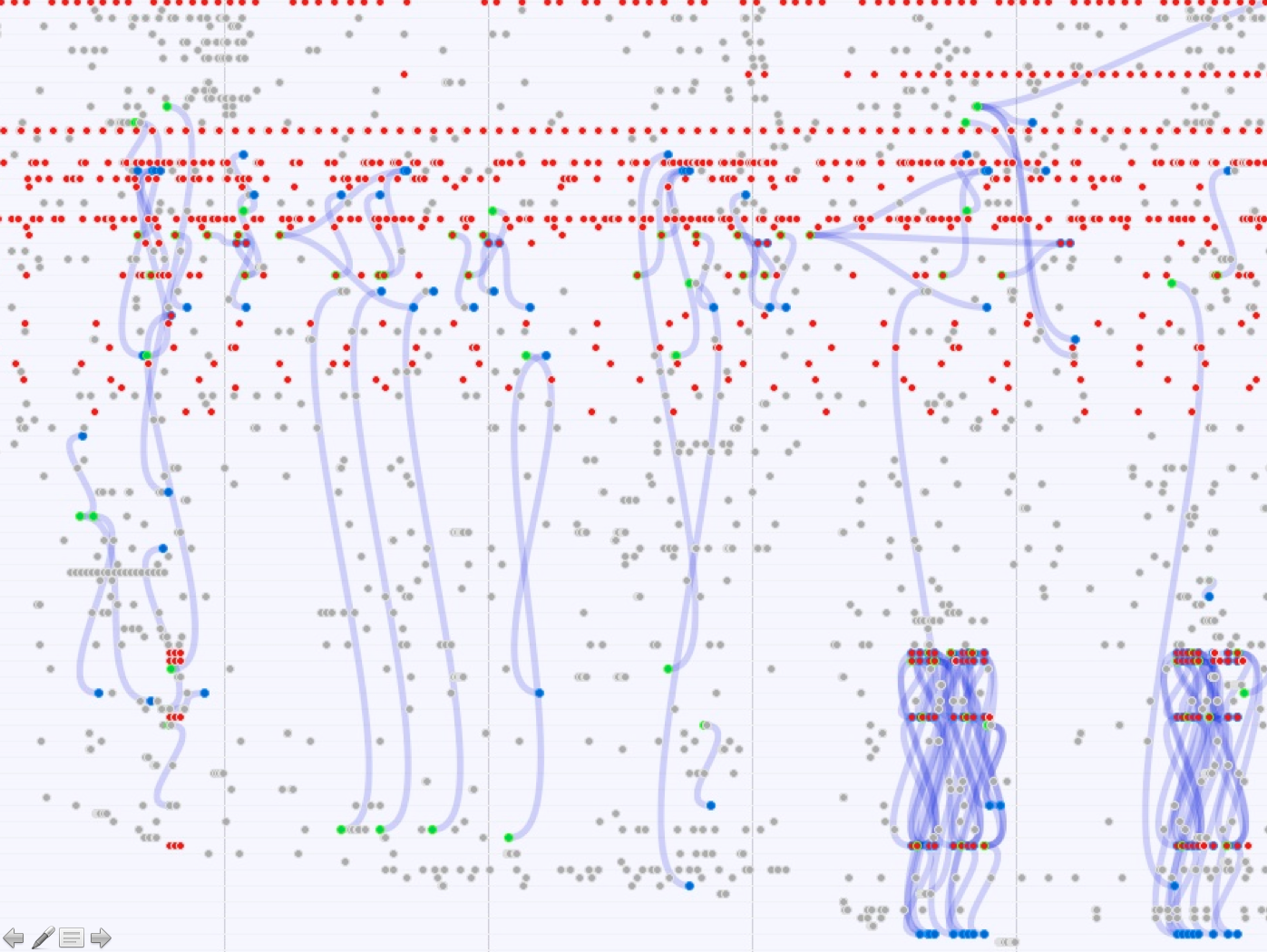
The second technique looks intro the re-occuring relationship between a particular user and a hashtag. The technique makes use of the Bi-partite graph user + hashtag in TCAT that can be opened in Gephi and arranged into two horizontal lines using filters. The heavy lines in the graph represent a very consistent, re-occuring relation between a particular user and a particular hashtag and are a strong indicator of bots. The results suggest that the hashtags that are strongly connected to bots transcend the upsurge in privacy and surveillance hashtags related to more topical, or short term events (generic hashtags in a sense, such as #whynot). Some examples of these highly dense relations between particular users and hashtags:
1. Twitter user: @Goodies_for_dad (over 50Ktweets) is highly linked with the hashtag parenting - the links in the tweets refer to products that can be bought.
2. Twitter user:@willsznet (over 50K tweets) is highly linked with the hashtag #sumedang, up until this day, the hashtag is used in the messages.
3. Twitter user: @SecMash (over 160K tweets), almost all tweets contain hyperlinks. The account is highly linked with #infosec.
4. Twitteruser: @lyricist_ces (over 9K tweets), all his tweets contain #whynot; very generic. This account actually @replies and all are the same text. An @reply bot. Interactivity to a large number of users and very different users
5. @privacy_law: (over 140K tweets), all tweets contain #law, used together with another hashtag #privacy. The Twitter account is linked to a website/blog. Taking a closer look the tweets contain hyperlinks to articles on these website. Here no addressivity is found. This is an alert/announcement bot
6. @gnudarwin: (over 140K tweets), linked with a website. Further investigation in the data set on privacy (TCAT) shows the sources of this users tweets are different: web, postling and bitly. Postling as well as Bitly, as a source, is a tool to help people to cross-post. So this is semi-automated tweeting.
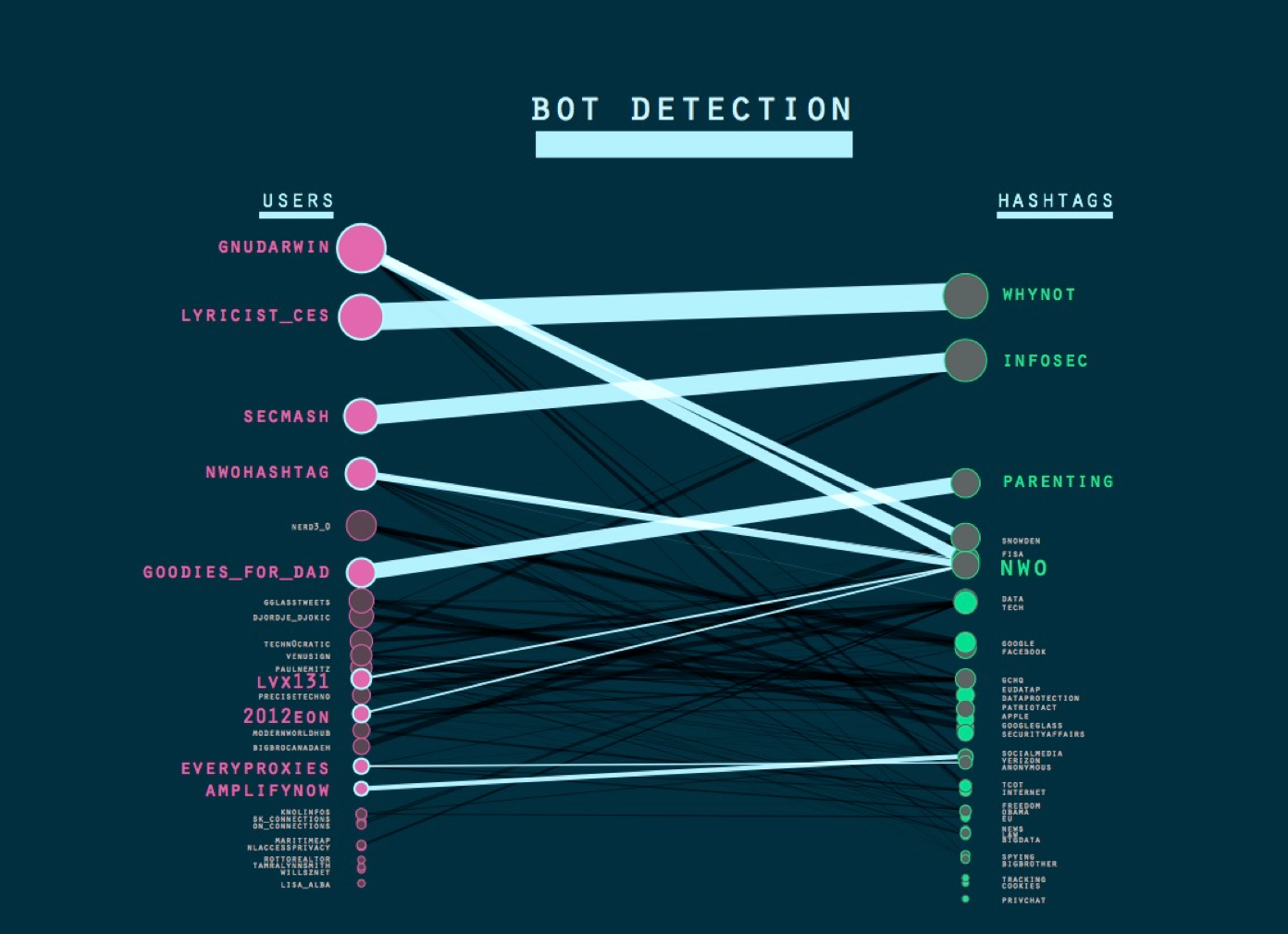
The third technique lists the top active users in the data set and we found the remarkable number of 16 out of the top 20 users to be bots. As pointed out in the User & Interactivity sub-project, the top active and top mentioned users do not overlap. There are however a few bots that are mentioned relatively often.
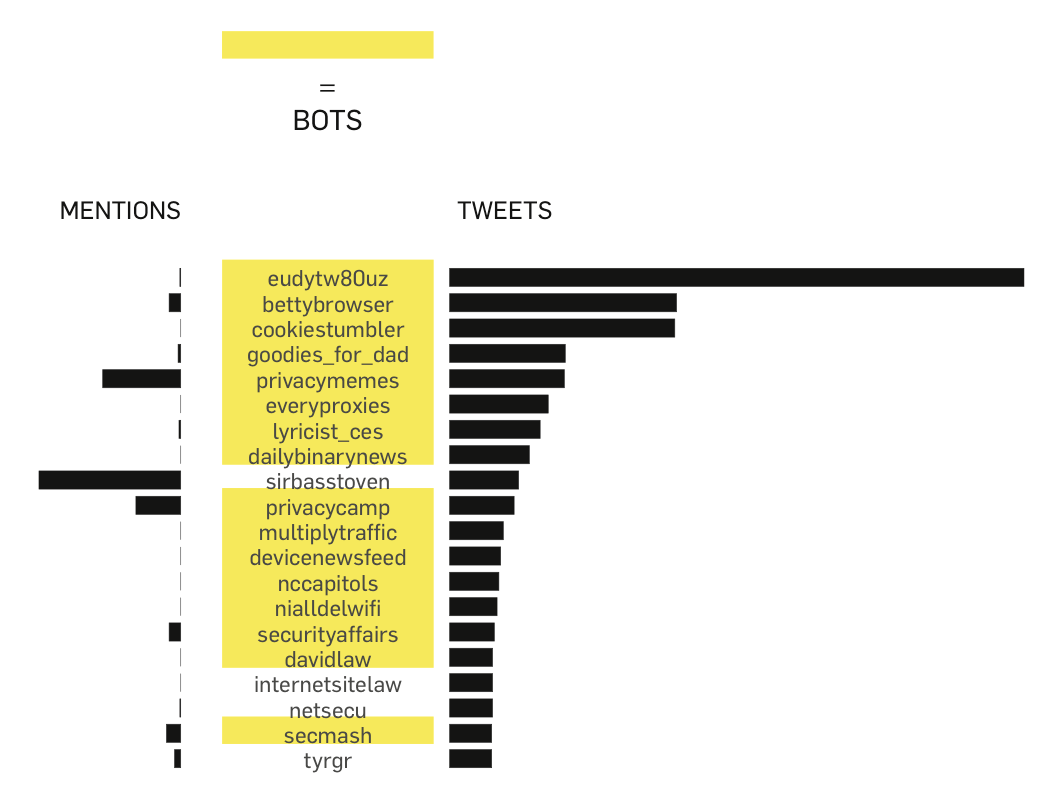
The fourth technique is by detection bots or bot like behaviour via the source aka device. There are multiple sources from which bots tweet and which are an indication of different types of bots or (semi)automated tweeting. Some examples include:
- cross-posting (twitterfeed), automatic diffusion from social media sites
- user-assisted (IFTTT, Buffer) classification and filtering websites
- magazine (Flipboard, Zine) App clicking
- button-clicking embedded SM buttons in sites)
Conclusions
Is a bot social? By focussing specifically on bots and (semi)automated tweeting, we seek to complicate the notion of the social by stressing that it is not just the human. Although it is not always straightforward to distinguish between a bot and a human user, it might be argued that the different techniques allow us to detect bots of various levels of sophistication. Some of the bots we detected are not very advanced, or put differently, not very good at blending in with the other users as their automated, machinic character is overtly clear. Whereas other bots display human-like behaviour or are interacted with and are much more difficult to distinguish from human users. Additionally, we found ranges of bottyness as some users are fully automated whereas other users are bot-assisted. This translates into different bot behaviours and therefor different signatures which can therefor not be detected with a single technique but in a set of techniques. Botty behaviour (e.g. high no of re-tweet, @replies, cross-posting) can howver be considered as a measure of the social as it implies an audience the expectation of uptake.
Our findings confirm the conclusions of the User & Interactivity sub-project that the high frequency tweeters are predominantly bots, or bot-assisted users, and has important ramifications for conclusions wbout social behaviour drawn from Twitter data without taking these users into account. Bot behaviour is characterised first and foremost by its repetitative and high volume behaviour possibly overshadowing other, more subtle forms of teh social.
Next steps
Develop manual indicators into automatable bot detection indicators.
Compare against other Twitter spaces (Climate change?).
Locate the bots (and their traces) in some of the other social measures.
III. Hashtag Profiling: How is a hashtag social?
Introduction
In our group work, we turned our attention to a arguably the primary object of Twitter analysis, the hashtag. Taking our cue from the wider projects intuition to treat the social empirically, we were interested in different ways in which hashtags can be said to express or materialize sociality. We wanted to find out how hashtags help to render the social explicit and to determine different ways in which hashtags can be used to operationalise the social. Thus, an active hashtag (one that suddenly gains high currency) and an enduring hashtag (one that is used consistently over time) might both be called social, albeit for very different reasons, and presuming very different understandings of sociality.
Research Questions:
1. How to extract/detect the social profile of a hashtag?
2. What is a social hashtag?
3. How is a hashtag social?
Method and findings
1. Hashtag Selection
Our first step was to select hashtags for further analysis. We were aware that in formulating and applying selection criteria, we were likely to make assumptions - implictly or explicitly as to what makes hashtags social. A focus on top hashtags can be taken to suggest that hashtags with high uptake are more social than others (thus approximating popularity and sociality). Conscious of the limitations we might thus impose on ourselves, we adopted two very different methods for hashtag selection, which could complement one another:
1. Enduring hashtags: common across intervals
Hashtags that consistently appear in the top 25 across all four intervals. These hashtags, we surmised, qualify as social, insofar as their uptake seems relatively independent from news events and other publicity spikes (endurance).
However, our findings mirrored those of the user interactions and bot groups: the top 20 was dominated by hashtags demonstrating low issue-specificity (there was for instance a large number of astrological terms).
The top hashtags per interval, then, were very botty, re-tweety, and newsy. We speculated here about the prize of success, and allowed ourselves a generalization: where there is high currency of terms (ie broad hashtag uptake) and accordingly a level of sociaity is attained, the consequence on Twitter is that the space degrades (generic and random bots take over).
Pragmatisically speaking, it is such degradations which compell us to test and generate alterative defintions of the social.
2. Daily Hashtags: para-institutional language
A second initial answer to the question how is a hashtag social suggests itself in the form of proliferating hashtags containing phrases and jokes (eg #overlyhonestmethods; #igetannoyedwhenpeople, #markmywords). These hashtags indicate highly informal - ie non-official, uses of Twitter, and as such may be taken as an index for social as opposed to institutional activity. A proliferation of such creative hashtags might be taken as an indicator of social activity (thus treating invention as marker of the social).
To produce an overview of inventive hashtags, we considered the top 50 of hashtags on each day of our selected period, retaining only those hashtags containing para-institutional language or neo-logisms. Plotting these hashtags over time resulted in Figure X and Y.
2. Para-instutitional language
Our two figures plot inventive hashtags over time: while the first figure (above) codes hashtags according to their frequency of occurrence, the second figure (below) distinguishes between entertainment, news, politics and pointless babble.
We arrived at these categories inductively, relying on exemplary tweets as guidelines:
ENTERTAINMENT
it's so sad that justin can't even have privacy at his home #givejustinhisprivacy
POLITICS
#IStandWithEdwardSnowden because I believe in the fundamental right to personal privacy.
POINTLESS BABBLE
#WhatIWant - Privacy. - a lot of money. - someone who will love me. - my parents to be proud of me. - high speed internet connection.
NEWS STORY
I am disgusted at the BBC's invasion of privacy. There must be a judge-led inquiry! #horizon #SecretLifeOfCats #horizoncats
In the second figure, note the burst in political hashtags once the story about the story of Snowden (the NSA leak) has broken, indicating a shift in the prevailing modality of hashtags in the privacy space on Twitter: from pointless babble to news and politics.
3. Associational profiles
We then turned to a tool in the making, the associational profiler (see for an introduction, our overview of WCIT hashtag profiles, made with this tool) to further examine our first set of hashtags, the enduring hashtags. The aim of this exercise was to further specify the ways in which these hashtags could be said to endure.
To begin with, we further narrowed down our set of stable hashtags for profiling to: privacy, google, security, surveillance, facebook. We excluded all hashtags that had low issue specificity (eg astrology signs), and all hashtags with low user diversity counts - meaning hashtags that dont have many users (thus adding a further marker of the social to our evolving definition). (further criteria?)
We asked: how are these hashtags inflected by the NSA leak?
The associational profiler tools applies methods of co-occurrence to detect the profile of a given hashtag: with which other hashtags is it frequently used and thus associated on Twtteri? Considering changes in such hashtag profiles over time, we speculated, offers a way to further establish their relative stability or volality. Hashtags profiles that remained relatively stable over time that remained more or less the same before and after the NSA story broke we thought, in this way further proved their relative independence from news events and other temporary upheaval in the political and publicity space. As such, they could be taken as an index of what we termed above the enduring social.
Intervals
We manually selected the following intervals:
week 1: 23/05-30/05:
week 2: 31/05-05/06: before the leak
week 3: 06/06-12/06: (from the moment of the leak to moment of tool malfunciton)
week 4: 13/06-15/06: (tail)
The hashtag profiles produced with the aid of the associational profiler affirm the enduringly social nature of our selected hashtags insofar as they present associations that are relatively stable over time. While in all cases, NSA/Snowden-related hashtags come to dominate the profiles in the 3rd interval, in almost all cases these newsy hashtags add themselves to the associations already present, without displacing the latter.
[..][expand]
eg Note tht the the profile of #infosec is an example of a privacy-associated hashtag that does not follow the pattern of the Snowden-burst. Instead, there seems to have discussion related to Judaism during interval 1, and another isolated discussion related to Verizon during interval 3.
4. The mundane social
In a next exercise, we stepped away from the assumption that the social should refer to the dynamics of hashtags (there stability or volatility, their proliferation). What we asked, if the social is to be defined in more substantive terms, ie in terms of the words making up hashtags? This intuition, while initially attractive, quickly appeared implausible to us.
What, after all, are the signature words of the social? We considered various options, and for a moment thought we had found a sound heuristic in fairly generic words like #photo, which might be used to worry about privacy in the mundane way we here appreciated as a stand-in for the social. As it happened, tweets associated with #photo were very botty. Surely, then, it is a highly contextual matter whether a given hashtags discloses the mundane? There are no clear signature words?
In the spirit of playful interrogation (Burrows, X; Reppel, 2013), however, we selected a number of words of which we had some hopes in terms of their ability to mediate what we termed the mundane social:
a) life
b) i, you, we : subjective words
(as prof Warren Sack during his 2-day visit to the DMI summerschool - helpfully suggested: professoinal discourse tends to be more impersonal)
[..describe figures below...]
5. URL profiling: newsy vs platformy hashtags?
In this last exercise, the aim was to determine the profile of hashtags in terms of the URLs. As URLs identify different types of organisations - news, social media, companies, NGOs and so on these could be used to determine the leaning of individual hashtags: are they primarily associated with social media, news or other types of organisations?
Thus, we asked, can we use URLs to detect newsy hashtags vs platformy hashtags?
We then saved our most literal answer to the question of what makes a hashtag social for last: If a hashtag was notably associated with social media URLS (twitter, facebook, pinterest), we now said, this may be enough to make it social.
Using the TCAT platform, we produced a URLs (host) and hashtag bipartite graph, and exported the graph into csv format.
We then downloaded all tweets in our data set and, on Google Refine, filtered by HTTP. We then retained all tweets with URLs, and extracted and expanded these URLS.
We manually categorized the URLS according to four organisational types (news, social, organisation).
Conclusions